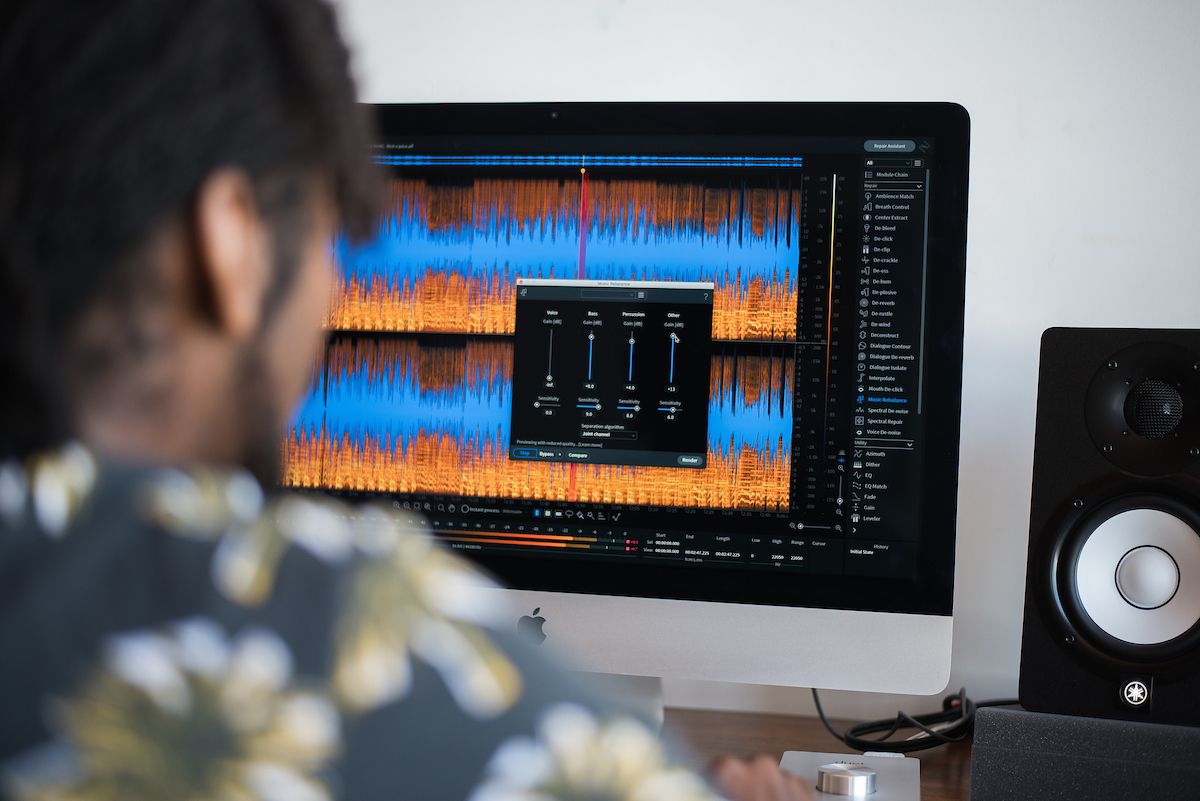
Exploring the Technology that Makes RX 7 Music Rebalance Possible
In this blog post, members of our research team talk about the new Music Rebalance tool in RX 7—what it is, how it can be used, and the source separation technology that makes it possible.
This article references previous versions of Ozone. Learn about the latest Ozone and its powerful new features like Master Rebalance, Low End Focus, and improved Tonal Balance Control by clicking here.
Here at iZotope, we are committed to developing cutting-edge technologies to enable people to create. Whether it’s Tonal Balance Control in Ozone 8, Track Assistant in Neutron, or other variations of our assistive audio technology, we want to build intelligence into our tools, with the goal of making it easier for people to be creative and do what was previously thought to be impossible.
The evolution of our intelligent audio technology continues with the Music Rebalance module in RX 7. Music Rebalance is a new tool that gives users the ability to boost, attenuate, or even isolate musical elements from audio recordings. It is a natural progression of our neural network-based source separation technology, first introduced in the forms of Dialogue Isolate and De-rustle in RX 6 and now evolved to extract multiple musical components from complex mixes.
What is Music Rebalance useful for?
Music Rebalance is an invaluable tool for any application where you want to quickly adjust levels of the individual musical components in a mix but don’t have access to the original stems. These applications range from practical to just plain fun. Mastering engineers now have the added control to re-level sub-par mixes without wasting time and effort coordinating file transfers with mixing engineers. Producers can sample music to their heart’s content, with the ability of turning down percussions so they don’t interfere with the drums in their beat, or completely isolating vocal phrases for their next remix. You can now utilize multitrack-like functionality on recordings captured using a single microphone. Music students can accentuate certain musical elements to help with their transcriptions. Even the karaoke enthusiast can remove vocals from their favorite songs, generating instrumentals that are as close to the real thing as it gets. Check out the video below for practical tips on how to use Music Rebalance in your own work.
How does Music Rebalance work?
Music Rebalance uses machine learning technology trained to separate different musical sources in a mix. Under the hood, Music Rebalance processes your audio through several neural networks, each trained to identify and isolate a particular musical source of interest (vocals, bass, or percussion). The outputs of those neural networks are combined to tell us the amount of a particular source present at each time and for each frequency of your audio. This information is used to adaptively filter the audio in ways that isolate a particular source of interest. Based on this separation, Music Rebalance then allows you to control the gain of each of these musical sources, along with a remainder source (which we simply call “other”), relative to their original amounts in the mix. This multi-network source separation is an extension of the single-source separation networks we released as Dialogue Isolate and De-rustle. In those modules, audio is similarly processed through a neural network trained to isolate a single source (e.g. dialogue and lavalier mic rustle, respectively).
The performance of our source separation technology is driven heavily by the data used to train our models. “Garbage in, garbage out” is the common saying, so we put considerable energy into making sure that we aren’t putting garbage in when we train! Each of the three source isolation networks (vocals, bass, percussion) was individually trained on isolated stems of that source type. For example, to train the vocal network, we first generated many custom mixes that consisted of vocals plus other instrumental tracks.
During the training phase, the network was provided each custom mix as input and that mix’s soloed vocal-only stem as an ideal output. In this way, after many training iterations, the network learns to output a mask that relates to the vocal components in any input mix.
We had to make sure that the training examples covered the scope of intended use cases for each of the trained networks. With vocals, for example, we trained on many different singers, each with different timbres and vocal ranges. If we’d trained on a single artist, our net would have gotten very good at separating that artist’s voice from the rest of the mix, but might not have generalized to separating *other* people’s voices from the mix! Similarly, we trained on vocals from many genres and on top of mixes generated from instrumental stems from a variety of genres, again to make sure that we didn’t accidentally train our networks to be good at opera at the expense of pop.
Additional training augmentation involved things like adjusting the amount of each source in the training mix, e.g., using the same vocal track at different levels relative to the full mix, so that we didn’t accidentally teach our networks to only perform the separation well when given a very specific type of mix.
What’s next?
Machine learning and neural networks have been readily adopted at iZotope, and we’re interested to see how they will continue to contribute to future growth in the areas of audio restoration, synthesis, and auto-mixing. The ability to separate individual instruments with Music Rebalance is an awesome first step into the future of neural networks for audio applications. It’s an exciting time in audio processing, and we’re looking forward to seeing all the new ways machine learning will be leveraged in the years to come.